Why need XAI?
With the aid of interpretability, people can better manage and appropriately trust AI systems, which is important for making decisions in the high-stake domains.
Where may need XAI?
Health Infomatics
People may need more interpretations towards health-related areas, including medical diagnosis, electronic health record (EHR), healthcare industry and etc.
Social Infomatics
More interpretability could also contribute to people's social life, and help to better detect as well as filter tons of spammers like fake news in social media.
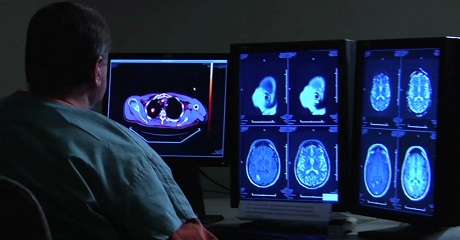
Medical imaging needs to show why and how a specific diagnosis come out.
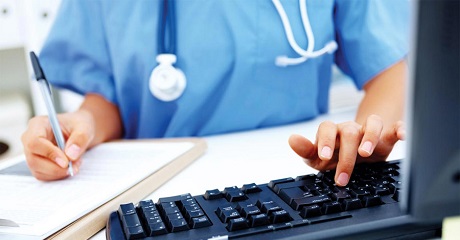
EHR could provide more evidence to people about the diagnosis and treatment.
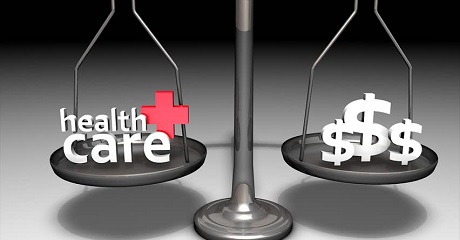
Healthcare policy system still needs more reasonable explanations to public society.
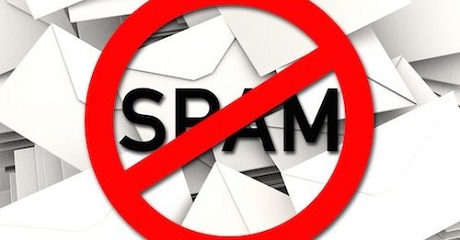
Better interpretability of spammer detector could help people to trust classification results.
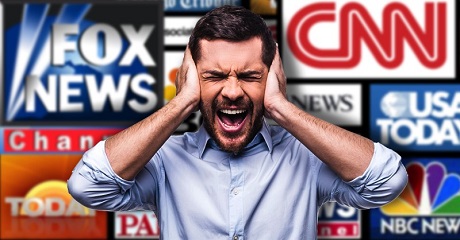
People need to identify the fake news with relevant evidences or justifications.
Our Current Work
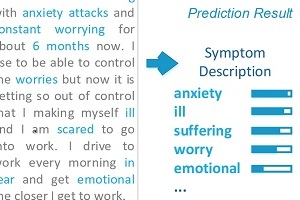
Our System can classify the health text into different catergories with interpretations.
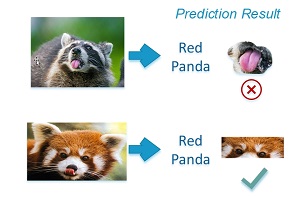
Our system can classify the general images providing with the corresponding reason.
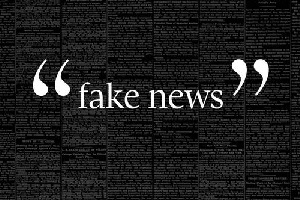
Our system aims to effectively detect and interpret fake news in social media.
Work 1:
Interpretable Health Text Classification
(Refining...)
Our interpretable health text classifier could help to process medical text, for example, in EHR. Each input sentence could be classified into 3 categories, i.e. Medication, Symptom and Background. Besides, the dominated features and discriminative patterns for each classification would also be provided as interpretations. Further visualizations are also applied for user-friendly interaction.
Work 2:
Interpretable Image Classification
(Refining...)
Our interpretable image classifier has two parts of functionalities. First, it can interpret the deep classification model by using some shallow models such as linear model and gradient boosting tree model. Second, it can interpret the predictions generated by deep classifier, and show the relevant hihgly-weighted super-pixels as corresponding interpretations.
Work 3:
Interpretable Fake News Detection
(Refining...)
In this work, we aim to detect fake news on popular news websites as well as social media, and provide different forms of interpretations such as Attribute Significance, Word/Phrase Attribution, Linguistic Feature and Supporting Examples. Further human studies are conducted to guarantee the effectiveness of our system in real cases. More improvement will be posted soon.
Publications
- Zepeng Huo, Xiao Huang, and Xia Hu . "Link Prediction with Personalized Social Influence", AAAI'18.
- Xiao Huang, Qingquan Song, Jundong Li and Xia Hu. "Exploring Expert Cognition for Attributed Network Embedding", WSDM'18.
- Jun Gao, Ninghao Liu, Mark Lawley and Xia Hu. "An Interpretable Classification Framework for Information Extraction from Online Healthcare Forums", Journal of Healthcare Engineering Volume 2017, Article ID 2460174.
- Ninghao Liu, Xiao Huang, and Xia Hu. "Accelerated Local Anomaly Detection via Resolving Attributed Networks", Proceedings of IJCAI'17.
- Ninghao Liu, Donghwa Shin and Xia Hu. "Contextual Outlier Interpretation", Proceedings of IJCAI'18.
- Mengnan Du, Ninghao Liu, Qingquan Song, and Xia Hu. "Towards Explanation of DNN-based Prediction with Guided Feature Inversion", Proceedings of KDD'18.
- Ninghao Liu, Xiao Huang, Jundong Li, and Xia Hu. "On Interpretation of Network Embedding via Taxonomy Induction", Proceedings of KDD'18.
- Ninghao Liu, Hongxia Yang, and Xia Hu. "Adversarial Detection with Model Interpretation", Proceedings of KDD'18.
- Fan Yang, Ninghao Liu, Suhang Wang, and Xia Hu. "Towards Interpretation of Recommender Systems with Sorted Explanation Paths", Proceedings of ICDM'18.
- Hao Yuan, Yongjun Chen, Xia Hu, and Shuiwang Ji. "Interpreting Deep Models for Text Analysis via Optimization and Regularization Methods", Proceedings of AAAI'19.
- Ninghao Liu, Mengnan Du, and Xia Hu. "Representation Interpretation with Spatial Encoding and Multimodal Analytics", Proceedings of WSDM'19.
- Yin Zhang, Ninghao Liu, Shuiwang Ji, James Caverlee, and Xia Hu. "An Interpretable Neural Model with Interactive Stepwise Influence", Proceedings of PAKDD'19.
- Mengnan Du, Ninghao Liu, Fan Yang, Shuiwang Ji, and Xia Hu. "On Attribution of Recurrent Neural Network Predictions via Additive Decomposition", Proceedings of WWW'19.
- Fan Yang, Shiva Pentyala, Sina Mohseni, Mengnan Du, Hao Yuan, Rhema Linder, Eric Ragan, Shuiwang Ji, and Xia Hu. "XFake: Explainable Fake News Detector with Visualizations", Proceedings of WWW'19 (Demo).
Try our online XAI fake news demo (current version) here!
If the link above doesn't work, watch our demo video on YouTube (Interpretable HealthText/Image Classification and Interpretable Fake News Detection )!
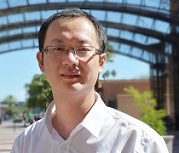
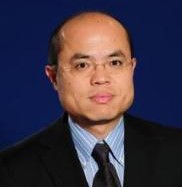
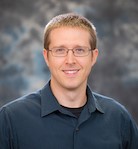
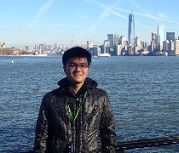
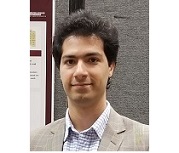
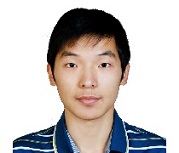
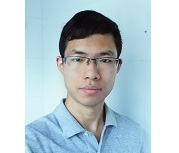
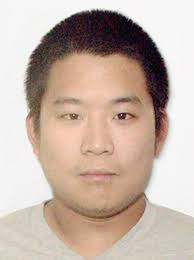
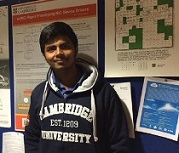
Contact Us: hu@cse.tamu.edu | 979-845-8873 | TAMU, 400 Bizzell St, College Station, TX 77843-3112
This project is funded by the Defense Advanced Research Projects Agency (DARPA)